AI and Security: Better Together
AI is transforming private and public sector organizations and security needs to be designed in. Host Wade Erickson met with Steve Orrin, Federal CTO and Senior PE of Intel , for an exceedingly interesting conversation on this topic on Tech Leaders Unplugged. Listen into an insightful conversation that you surely won't want to miss.
In this engaging episode of Tech Leaders Unplugged, host Wade Erickson dives deep into a thought-provoking discussion with Steve Orrin, Federal CTO and Senior PE at Intel Corporation. Exploring the profound impact of AI on private and public sector organizations, Orrin stresses the vital importance of integrating security from the outset. Tune in for an enlightening exploration of AI's role in reshaping cybersecurity.
Key Takeaways:
- AI's integration reshapes organizational landscapes across sectors.
- Security must be inherent to AI design and implementation processes.
- The conversation highlights the necessity for proactive cybersecurity measures in the AI era.
Wade Erickson (00:13):
Welcome all to another episode of Tech Leaders Unplugged. We are excited to have our guest today Steve Orrin. He is the federal CTO at Intel, and so works on the public sector side of Intel with the federal government projects and such. Welcome Steve to the show, and thank you so much for spending time with the team the, with the audience here. And so before I get in, we're going to talk about AI and security better together. You know, we've had a lot of shows on here about AI and the blending of security is a big concern, and I don't think we discuss much of that in, in in our society at large. So Steve's going to look at how, talk about how he's been approaching that and appreciate your involvement with our audience here. So please introduce yourself a little bit. I'm sure everybody knows who Intel is, but maybe talk a little bit about your port part of Intel and where you focus your time.
Steve Orrin (01:20):
Thank you Wade, and thank you for having me today. So as you said, I'm the federal CTO of Intel Corporation. And, and in that role, my job is to help the federal government and the broader public sector understand and adopt technologies, both what's available today as well as what's coming in down the road and help integrate those technologies, those architectures into mission and enterprise systems. So a lot of it is working with the ecosystem, the large primes, the other software and hardware providers and OEMs to help get the technology enabled so that the federal government and the public sector can take advantage of the latest and greatest technologies, can affect the mission, can improve and make more efficient their enterprise. And then the other part of my job, which is then translating what the government needs back into Intel so that our products and technologies can better meet those requirements and needs both today and in the future. So it op it affords me opportunity to spend a lot of time with the customer and their ecosystem as well as the business units and what's being developed and in between get to do some interesting innovations to help form, fit and and customize products to meet those government missions. As you mentioned, the topic we were going to talk about today is around AI and security better together. And the way you want to look at it is, there are two sides of the coin on the one side is how do we secure AI? And the other side, which we'll get to in a moment, is how can we use AI to better secure other things, to do better cybersecurity and and risk management? So let's start with the first one and unpack that one a little bit of how do we secure AI? And one thing to understand is that, that AI isn't just the thing. You see the really cool, you know, chat bot or object recognition system or recommendation system that you're using online. There's a lot that goes into developing, building and maintaining that AI based system and that whole pipeline from data sourcing and data collection and data generation through the wrangling and curation and labeling and the tuning and model development. Every step of that process is a, an opportunity for something to go wrong, for someone to attack it, for a vulnerability to be exposed. And so when we look at how do we secure AI, we have to look at the entire life cycle. And so it starts with getting better visibility into how the AI is built, how that system is being deployed, building in controls along the way to make sure you get deep analysis of, well, what are the data sources? And this is not only important for security, but it's also important for the ethical and responsible use that everyone is talking about. How do I build a trustworthy AI if I don't know what's in the box? And this theme that we are hearing over and over again, whether it's around supply chain security and everyone's focused on software has to apply to AI as well. How do we get better visibility into the building blocks that are forming the foundation and the systems infrastructure and data that is driving these AI solutions? And so it starts with making sure you have good practices, coding methods, as well as understanding of your sourcing and of the, of the tools you're using and of the decisions that are being made when you start integrating the AI. So like I said, we have to start with having a secure foundation and how we build these AI systems. And then we have to look at how do we protect the systems themselves when we're deploying them. These AI's are running on infrastructure rather than the cloud on edge devices. Oftentimes they're being used for in, you know, interesting use cases of recommendation or object recognition, but a lot of times they're being used in very serious, very mission critical applications, whether it's making sure your car doesn't run you into a tree, giving recommendations to a doctor about what procedures to perform, or in the case of the public sector and government, it could be making life and death decisions and understanding, you know, where I need to go to prosecute the mission. So ultimately we have to build trust, not just only in the AI, the infrastructure that that AI is being deployed into, and that's being able to protect it from poisoning and attacks on the AI system itself, as well as the systems upon which it's running. We need to have the ability to have a foundation with secure boot technologies, confidential computing data, encryption, data protection throughout the lifecycle. All these things that we sort of take as part of normal security processes need to be applied to the AI systems. The one thing that makes AI a little different from just playing a web server or transact an interior application server or even an app onto a phone, is the complex distributed nature of AI. And then the fact that the AI isn't a static thing. So when take into account those two aspects, and one, there's oftentimes you have an edge sensor that's collecting or, or, or generating data that's being used to be able to funnel into the AI system. And then an output, whether it be a recommendation or an action is being performed based on that ai, that complex system. It's an end-to-end scenario where we have to look holistically about how do we secure all parts. 'cause If you focus just on the inference engine, which is important, but don't think about, well, how am I protecting the sensors to make sure they're not perform, providing bad data or someone isn't poisoning the data pool that's feeding that AI, then we're missing the boat. And so you have to look at it across the entire lifecycle and the entire deployment scenario. The other aspect I just mentioned is that it's not static, especially today with generative AI and large language models. These AI's are constantly evolving and constantly learning. And you know, if you went to DEFCON this last summer, you would had entire trek dedicated to hacking AI. And many of those attacks are poisoning attacks and impro injection attacks on the deployed system. And the reason is, is cause the AI is, you know, in some sense a living thing, it continues to evolve. And so if I'm poisoning data, if I'm putting in injections into their queries, I can actually skew the AI in its learning based on what, what's being injected. And so we have to be able to put the right filters both in on what's being injected in, but also monitor the AI to make sure that it's continuing to operate within the scope of what we define as good. And that's hard. 'cause a lot of times we don't know exactly what's good for an AI as far as its outputs because you don't know until you start querying it. There's a lot of really interesting research going on right now of how to keep your AI from hallucinating or potentially forgetting information based on what it's learning and it's testing it. And that's really the kind of processes. So I'm not saying there's one technology or another is better, but it's making sure you have a process where you can validate that AI is still valid and trustworthy post-deployment. And these are the kind of controls that we want to start thinking about as we deploy these enterprise scale AI solutions. So again, it's about the life cycle and about securing the, the holistic approach of how we deploy AI with the right tools, processes and techniques with monitoring. And if you peel back all of that, it comes down to visibility and having a risk management approach to your AI, which doesn't sound that different from any other cybersecurity activity. It's, this is a lesson we continue to learn, you know, whether it was the, the web introduction cloud mobile devices, we have these applications deployed and then we think, oh wait, maybe I need to secure these things. AI is the same thing. We need to start building the security in from the get go because it's going to be very hard to bolt it on after the AI has left, you know, left the building. And so that's why building in the right controls, processes and procedures, getting visibility through each step will help us downstream be able to be validate the AI, make sure we can trust it, and then communicate that trust to the relying parties. Cause the other thing, and then we'll get to the last part is that oftentimes you build an AI in one organization, but the one who's actually getting the benefit, who's using the AI is a completely different organization. And so while the, the company who built it may have good visibility into how they built the AI, the consumer of that AI, the customer for that AI is the one who ultimately has to make the decision, do I trust it? And so there needs to be a relationship, a way of attesting to an AI system. Are you trustworthy? And the way you build that attestation is by having the, the developers and the providers of that AI have that visibility that then they can provide through an attestation to the relying parties. So we've spent a lot of time not talking about that one side of the coin, how do I secure AI? And it's an important part of the puzzle. But the other thing to consider, and this is something where there's a lot of hype around it, but not a lot of practice yet, is that AI can absolutely help us in our cybersecurity and our risk management. It is a powerful tool that is only now really starting to be applied to these domains. Now lots of companies out there have been talking about how they use AI for their advanced detection and prevention techniques, and there are some really interesting innovations out there, but I would say the vast majority of them are really just doing pattern matching on steroids. They're doing it, you know, machine learning, some advanced pattern matching and really speeding up what they were always doing the, the day before. Where we're seeing a lot of promise is using some of the more advanced AI techniques to help detect, to do early detection by being able to do similarity analysis, be able to do behavioral analysis, predictions for what could happen based on what we've seen happen. And that's where a lot of the research is going. But one of the things I want to posit real quickly is that the biggest benefit that we in the cybersecurity industry could get from AI is not having a really cool, you know, shiny object that we can deploy as a next generation sensor. Well, there will be lots of those developed. The biggest benefit for enterprises and organizations for their cybersecurity was be taking the AI and the automation that it can provide and applying it to their existing infrastructure to basically take care of the 80% of the stupid stuff, the firefighting, the constant patch management, the constant whack-a-mole of, oh, I've got another hit here, I've got another signal there. And today you're using your, your precious few underfunded and overworked cyber talent to go track down all of these things and to go do patch management and try to keep up with the CVE databases and then the supply chain and asset management to be able to correlate. Those are the kind of functions where AI can actually shine and where you can actually automate the process of identifying if you have the vulnerability based on CVE and supply chain, be able to do automated testing and correlation and automated patching using the AI and machine learning systems to do that, to wipe out that 80% and then let your really, like, like I said, your underwear, underpaid, overworked team focus on the 20% hard stuff, the interesting problems that there's, you know, one example of, so an AI can't train on that. Great. Put the human on that one and let the AI handle the rest. And I'm not saying we're going to replace all of our cybersecurity talent with an, with an advanced AI, but we can reduce their workload, reduce the firefighting, and enable them with AI to be more effective in their jobs. And what companies will see is a huge return on investment for the use of AI internally by applying it to these more manual and, you know, repetitive processes that they just have to do every day. And that will make them more efficient on their cyber and also allow them to get rid of a lot of the vulnerabilities that are still hanging out in the organization because there's only so many hours in a day that a human can go and patch a system or track down a firewall hit. And that's where we can get the biggest bang for the buck on using AI for cybersecurity. The last point I'll make is that there's a lot of companies that are hesitant to use AI for cybersecurity. They're like, well, it's not trusted. I don't know enough about it. This is too new. I like what we're doing today. But let's just be clear, the adversaries are absolutely adopting AI today to make their campaigns more successful. They're using it in advancing phishing campaigns in doing much more higher fidelity information disclosure as well as reconnaissance. They're using the tools against us today. And so it, it's, it's only at our detriment that we don't start to take a more proactive approach to using AI internally. And, and to finish off, one thing I'd like to leave people with is that oftentimes they're hesitant to build automation and these automated capabilities into our cybersecurity is, well, I have to be worried about breaking something. Well, the problem is, is that we have to be okay with the CEO losing email for 30 minutes to prevent a data breach. And I think we have to get past that, that hump to allow us to automate these things, to use the AI to identify and correlate and patch automatically. And if it breaks something, we have resiliency in our networks, we have failover, we can recover. But when it comes to things like ransomware and data breaches and these stealthy advanced persistent threats, the amount of time, energy, and money lost far outweighs the, the, the minor inconvenience of a system going down or a website coming offline. And so again, it's really about looking at AI and security from both sides of that coin. How do we secure the AI's that we're building and deploying, and how do we get better trust in them? And then how do we use them to make our systems more secure, our environments more secure going forward to meet the adversarial threats that we're dealing with today?
Wade Erickson (14:29):
Great points there. One, I really enjoyed the how you, you, you press the issue that these technologies, these AI is really to assess pe support people. So that man plus machine kind of model where yes, we are going to, you know, be able to push some of this more mundane stuff and some of of the stuff that actually slips through because it is mundane. People want to work on the exciting stuff so they, you know, don't get a chance to stay focused on that and let the machines help with that. And then the second thing is, you know, I think back to all the, the phish training that we have, right? And how much of that focused on mis you know, bad spelling, bad grammar, as if it was written by somebody from a foreign country that doesn't have a good command to English or something. Well guess what? ChatGPT and all that bill. Beautiful, well formed sentences. And so just as simple as those phishing emails, you, you have to change your phishing training 'cause that stuff's going to go away. I'm sure it has. And these, these are getting just more sophisticated and just shows how the, the, you know, the bad players, the bad actors are using the AI against us as well. Where we were using it to write all kinds of good documents. They're, they're using it to attack us. So well great, this great, great content. And then as we kind of think about AI, of course in the news there's been some talk about the ides that are helping to write code. So you have developers that are AI and able developers now. And of course if 80% of the code is written by the AI, you know, one, I I wonder how much they're going to check that because it was learned on something that you don't know unless it's your own internal code. So it could be embedding bad scripts in there. Two, you got to have the expertise to even look at the code to know. And so my concern is that developers are getting lazy on the code, you know, just like they're cutting and pasting libraries and you know, code, code libraries and stuff. How much did they really look through that code to see if there was some bad stuff embedded in there so the AI could insert it? We already have that problem with libraries and stuff like that. So again, AI is, you can't let AI just run on on its own. I've always had to fix the things. And that really comes from expertise. And people think, you know, and as people get less and less expert in their job, 'cause we didn't have the AI around to help us with our jobs, so we had to build this expertise on how to look at something, right? So that's just some of the things I think about. So it aligned with what you're thinking about. So let me jump into some quick questions here. So as you are developing products there, and I think about your customers and how they're feeding, you know, are they asking for this or is it you just being smart about, hey, when we build products, this is something that worries us, you know, is are the federal customers asking for this? You know, and how do you approach the integration and machine learning in, into the technologies and products that you're building based on that user experience and those kind of things? Or is it you're having to push that yourself?
Steve Orrin (17:43):
So Wade, I think it's a little bit of both. We the federal government and public sector, like every other organization out there is absolutely seeing this shiny object of AI. They see the potential of what it could do. And so they all want it. They all want it somehow. It's really, we're seeing AI being looked at both in lab as well as in deployments across the board. Everything from, you know, obviously DOD use cases to civilian use cases. It's, you know, object recognition has actually been used for a long time in a lot of places to help identify, whether it be, you know, USDA or forestry to look at you know be able to scan large swaths of forests to be able to look for blight or in the case of Homeland Security, be able to do quicker identification to speed people through TSA or customs and border patrol. So we're already seeing the use of AI, it's not the large language models that everyone's, you know, excited about today, but it's already been deployed. And there's a foundational thing that in government that it needs to be secure in order to be deployed. They don't always understand what that means. There's the baselines, like the NIST recommendations about how to deploy applications and systems into the government, the what's called the 853 guidance. But we're also starting to see guidance from, from NIST and others on how to deploy proper AI and trustworthy AI. And so we're starting to see the beginnings of the requirement as we move into more mission critical applications. And again, both on, you know, in the VA for healthcare and in the DOD and other places, there is absolutely a strong requirement for security. Again, they want the security, they want to be able to trust it, they need to be able to protect the data because of the classification levels as well as the sensitivity on both sides, you know, civilian and on, on the military side. But they don't always know what it takes to do that. And that's sort of where the interface between industry and government really shines. They have the requirement, industry has the sets of technology and it's really about bringing those two together to identify, okay, here's how you're going to deploy your ai, here's what you're trying to solve, the, what's the actual problem set? And then looking at what are the right technologies and processes that can be brought to bear to help secure it to the risk profile you need. So to go back to your question, as we listened to the requirements around data separation and data protection and the government being able to do both high side and low side operations with an AI and the aggregate problem of when you pull data together, even at the unclassed level, it can raise its classification or in the case of PII, you know, little bits of information quickly become PII. And when you have an AI that's just consuming everything, this becomes a real challenge. We look at what are the kinds of technologies that we can bring to bear both from Intel and with our ecosystem that can help with that. And so technologies like you know, confidential computing is probably one of the hottest ones right now. Being able to put your AI into a protected container that is hardware controlled, encrypted memory, so that the actual inference, the actual training, the actual data management can happen in a protected space and the testable protected space, whether in the cloud or on in the data center or on the dev device edge device. And that can give you those security controls, that security monitoring for the application for the ai, so you can maintain the control throughout its lifecycle. So helping to get those technologies adopted into those use case is really where industry at large is working with the federal government and I'm sure with other regulated industries to help them dump the right, adopt the right technologies to meet their, their enterprise and mission needs and requirements. And it's a two way street is understanding the nuance of those requirements and the government has some very unique ones. It helps to us to build technologies that are, that fit a much broader set of customers. Similarly, as we deploy these technologies into the commercial space, oftentimes a lot of what I do is what I call federalizing commercial solutions. Something that may have worked for a logistics company to be able to protect their data as they're delivering information to their constituents is just as useful for the government when they're trying to do logistics management and supply chain management. And so taking those commercial solutions and being able to then apply them to the federal mission. And so it is that two-way collaborative streak about how we communicate back and forth the requirements and the capabilities.
Wade Erickson (22:04):
So you know, as I think about building these products obviously AI and the speed at which individuals are absorbing this, like you said you know, a lot of early conversations around AI is about, you know, what is the landscape of solutions and, and what makes sense to apply to the problem. And then second, you know, if we're thinking about security, which ones are more sensitive to a security issue, if we did int fact adopt that AI, you know solution into our total solution set, right? So, you know, how do you, you know, when you have your team members, 'cause right now we can't assume everybody's completely AI understanding right there, you have pockets of that. So tell me a little bit about, you know, how your teams from a collaborative standpoint have leveraged some of those insights in a successful manner. Because I think a lot of companies that are looking at putting AI in, you may have only one or two people on the team that really kind of understands this stuff and the others are just, you know, hey, they're just trying to catch up and, and so, you know, tell me a little bit about how you kind of balance that with, you know, folks that are, I mean, do you have to put 'em on multiple projects? Are you guys building now the gurus of AI and inserting them in pieces, or are you really training people hard to understand this stuff and catch 'em up?
Steve Orrin (23:37):
So Wade, you bring up a good point and part of what makes a successful team in these spaces is having that diversity of knowledge. So I absolutely have some absolute AI experts on my team and some rock stars on different aspects on algorithmic development on one side as well as enterprise scale of data systems on the other. And then, you know, one of my people is a performance guru. She knows how to craft an AI solution that can get the best performance and get and be able to handle the large data coming into it and pairing them in the projects with security experts, with networking experts. I have a person who's a firmware expert. So pairing them together on projects that cross chaining happens when you bring people like like-minded individuals together with diverse problem sets and diverse experiences. And you put them at the problem. And let's face it, no one has solved every problem, but they have what they've done and their experience and their skills and that need to go figure out the next big problem. And so part of it is that cross training that happens, right? Naturally bringing together those teams and having them work on actual solutions. I think from my team in, in specific, one of the key things that's been really successful is making sure that we have practical problems to go solve. A lot of times products are developed on the esoteric, there's a product manager came up with a list of requirements, they toss it over to the VP of engineering and the VP engineer says, oh, we got to build this product to beat these 16 things and get it out the door in a quality way. And the successful organizations actually have engineering and product management and many times bring the customer in so that they're all in the loop on what you're actually trying to do. And so one thing I've been try I do in my organization is when possible bringing my architects and my engineers to customer meetings, even though they're not there to present, to listen, to hear the nuance of what the problem is. And oftentimes they'll pick out something the customer didn't even realize they're, they're going to have to be challenged with because they understand the technology or some of the limitations of the technology. And so getting them exposed to the actual end customer or to the ecosystem provider that's going to host that system is impm is absolutely imperative for that cross that true collaboration. So again, it's having a diverse team that can work together on projects and not leave people in silos. And then getting both sides involved, having the, the customer involved in the engineering conversations, having the engineers come out and hear the initial customer requirements go on site and see where this thing is going to be deployed. And you know, one time we had a interesting use case where we brought the engineering team out and they looked at the problem and we're talking about it and then they took us a tour of their data center. And one of my engineers said, you know, that all this stuff is moot because they don't have enough power to power the servers they need to actually even deploy this AI engine. So there was a fundamental problem, no matter how cool the AI problem was, they didn't have the power in the day. So they had, you know, the customer realized that and suddenly they were, you know, they had to do another part of their project, which was upgrade the power for the data center. And so those little things are kinds of things that both sides can learn from that collaborative environment.
Wade Erickson (26:41):
And, and I think that's exactly the case as we're learning into these new territories, somebody has to pave the way and you know define that path. And so these kinds of things will start to show up in checklists and other things. Like you said, the power, make sure you got enough power because these are big power hogs and you know, and heat and cooling and all that stuff, you know, so alright. Well I wanted to pivot at this point, you know, as we're kind of getting near the time, I like to pivot this part to talk about you and your career. A lot of our watch people that watch the show, you know, would love to get into the c-suite and you know, you had a a a bit of a unique path. Your, you you, your background was largely in security and you progressively grew and, you know, been with Intel for 19 years. I mean that's, it's getting more and more rare that people are with companies that long and you were able to navigate your way all the way up to a CTO and that is not easy to do within a company. So, you know, tell me a couple of the key events that you can remember over this last 20 years or so that maybe helped you know, get you unstuck from the pack so that you could be noticed and appreciated for what you do to be invited into that level of being a CTO at Intel.
Steve Orrin (28:01):
Wade, it's a really good question and I, I want to go back a little bit even further. So I started out as a startup, CTO doing startups throughout the nineties and two thousands and had some great mentors that helped me along the way to get you know, at each stage of my career development when I got acquired by Intel in 2005 I didn't expect to stay there very long. I was like, well, I'll do my six month sentence like any startup, you know, does when they're acquired and then go do another startup. And at the time, the, the head of the software group who went on to become president of Intel, Renee James came to me and said, Hey, I'm building a pathfinding team. Would you like to lead security pathfinding for Intel? And I thought that would be an interesting opportunity to basically play CTO with Intel's budget. No VCs. That sounds like a great thing to do for a few years. And what it afforded me was the opportunity to really start to look at the broader technologies that Intel has to bring brings to bear and innovate on software and firmware on top of that hardware to do novel things in security. Some of the key things I learned along my way and I learned very quickly is that, you know, being in a startup, even a very successful, you know, you know, medium mezzanine level startup is nothing to pair you to playing big company like at the scale of Intel. So I had to learn how to play big company and a couple of things I learned very early on in those first couple of years is, number one, you need to, you know, go out and network. You talk to people, find out who you know, basically who are the people and where do you know who are the ones that know where the bodies are buried. You want to find those key people who are influencers and also ones that are just, you know, really good at, understand the technology and get them on your team. And it goes back to something I learned early in my career. I had this great opportunity to meet with Jim Collins of the Good to Great fame and he sort of presented, you know, his information and was very instrumental. And one of the things that have always stayed with me is that get the right people on the bus and listen to them. And I think that's, whether it's on your own team or on a matrix team, is making sure you talk to and learn from the smartest people and know that there's, you want to have the smarter people than you together on your team, whether it be your actual team or the broader matrix. The other thing that I learned probably about four years in as my first product started to come out and we were ready to start to, to get it into the production, you know, into the plan of record is understanding both the value chain. What is your feature, your widget, your product, who actually benefits what in customer, what, what use case, what, what channel partner, who is the, the one that gets the value? And then from playing big company, who are the key executives that care about that value creation? 'cause Oftentimes your executive cares about you getting your product out the door on time period, end of story. But it's a different executive that actually owns the customer or owns the product that you're going to land on that and you're opening up new markets to 'em. So understanding that value chain and then working with that executive or that director and helping them succeed. So when you make them shine or you give them opportunities to go after or grow their market, that's how you know, you become a star to them and then they become helpful to you as you try to go do new things. You that past performance and proving that and being able to understand that value chain. And that's been useful throughout my entire career at Intel. 'cause It also helps you communicate the value of what you're trying to bring. Security is often hard to say, well why should you add the security widget? Well you're going to prevent this kind of attack. Who cares? Really the way you have to try to describe it is it's going to allow your customers to be, you know, to meet these regulatory needs, which then allows them to deploy your, your widget, your product and be able to meet the regulatory needs at the scale they want to and continue to there. That's how you under, you know, communicate that value chain and be able to talk in business terms, not in, you know, cross-site scripting, SQL injection and buffer overflows. 'cause You'll lose your executive audience instantly if you go down this that track. And so it's really about how do you make, how do you communicate what you're trying to accomplish? Because at the end of the day, I built some products that prevented certain kinds of attacks, but it's really about what does that enable and who cares about enabling that with some of the key things. And then the last thing is when you build your teams, one of the things I found that Intel's really good at is that if you build a good team and you make them successful, you will rise accordingly. And so having engineers that go on to become directors, having engineers go on to become senior PEs and others, that when they look at you and say, well what have you done for Intel? It's not just what product did you get out the door, but how have you grown Intel's teams and Intel's people? And so by growing my people, I'm in turn I'm doing a benefit to my career. 'cause They say you are a person that grows teams or that makes people successful and helps them. And that is one of the key aspects I know at Large Corp, you know, technical corporations is really valued is how do you grow your teams and how do you scale them
Wade Erickson (32:50):
So I hear a lot of servant attitude there. I get people on your teams that are going to provide the greatest value, focus on what's going to be support your you know, executives above you to show them that you're providing them value to make their life easier and more successful. And in time they want to bring you along. And that's, you know, that's you know, great advice for folks and really put your ego aside. It's really about taking care of other people and the more you take care of other people, the more you have a servant attitude towards your leadership, your company and your teams, the farther you're going to go. And yeah. Great, great, great points. Appreciate that. So I think we're at the top of the hour these shows go so quick. want to quick introduce this and then we'll say goodbye to folks. So next week our show is with David Mully, CEO of lodge IQ. It's on Wednesday the 15th. Lodge IQ is a travel tech company and the topic's going to be unified commercial strategy is in vogue, but the tech continues to silo. So talk a little bit about the travel industry, tech involved with that. And yeah, on Wednesday the 15th, again always 9:30 Pacific time for the live show. Catch it as a recording after. Alright, Steve, thank you again so much. This is a really a timely topic area. You know, I think security, when you do security good, guess what? You don't get noticed, right? And that's a lot of folks, you know, it's only when you screw up security is that you get noticed. And so it's hard to sell and you know, hats off to people like you that have pushed and fought and got this stuff in there because again, people don't know how solid your army of security is until it's breached, right? And, and then you look for people to blame. So you're in a tough space at a fantastic corporation and we appreciate you spending time with us and giving you insights on.
Steve Orrin (35:01):
Thank you Wade.
Wade Erickson (35:02):
Things are going
Steve Orrin (35:03):
Yes, thank you very much. All right,
Wade Erickson (35:04):
Awesome. All right, until next week have a great weekend and enjoy the enjoy your week. Bye.
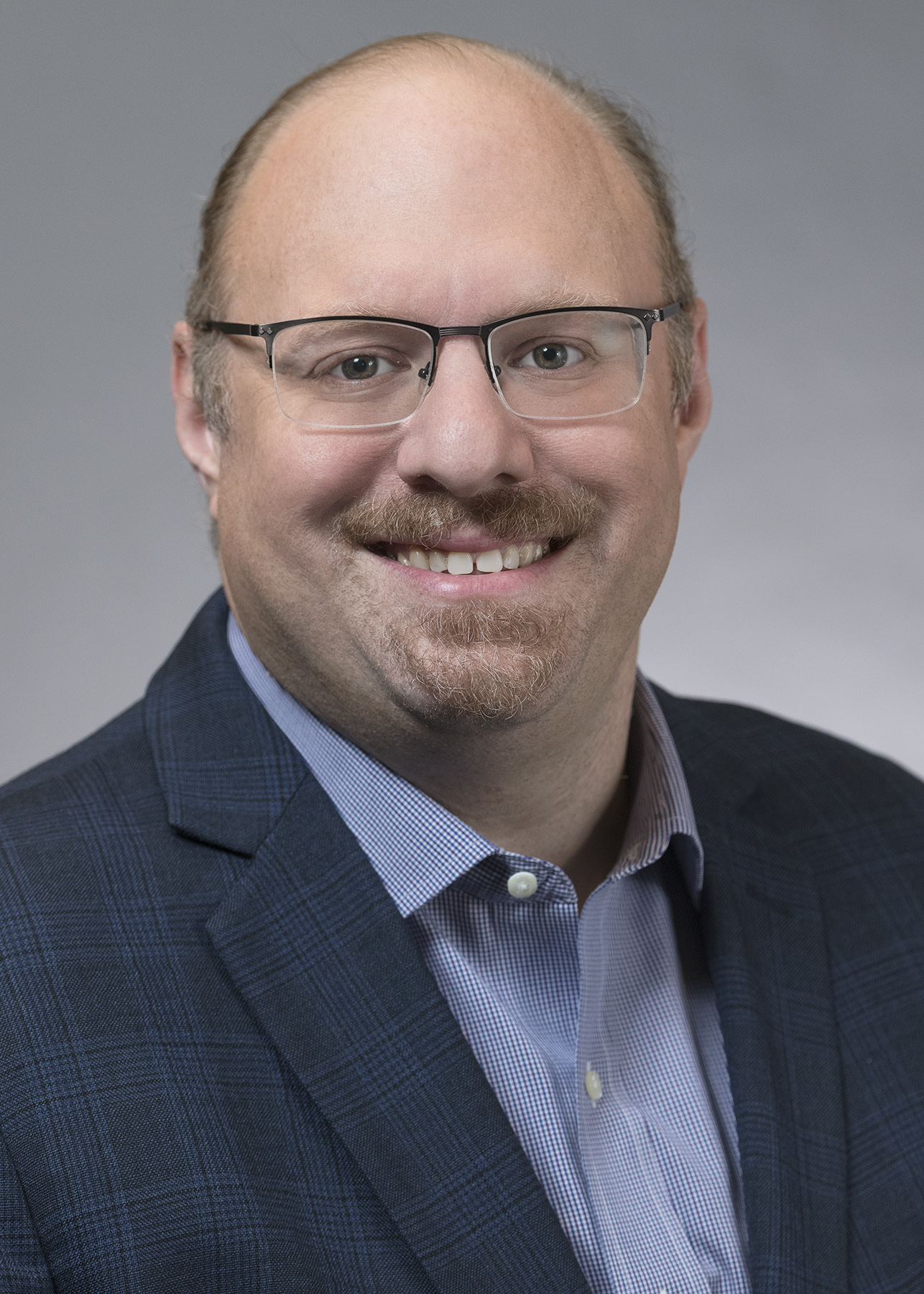
Steve Orrin
Federal CTO
Steve Orrin is Intel’s Federal CTO and a Senior Principal Engineer. He leads Public Sector Solution Architecture, Strategy, and Technology Engagements. He has held technology leadership positions at Intel where leading cybersecurity programs, custom hardware and software architecture and solutions, products, and strategy. Steve is a cybersecurity expert and sought-after advisor to public and private sector leaders on enterprise security, risk mitigations, and securing complex systems. He is also a leading authority on Public Sector/Federal mission and enterprise systems and solutions regularly engaging with United States government senior technical and mission leadership.
Steve was previously CSO for Sarvega, CTO of Sanctum, CTO and co-founder of LockStar, and CTO at SynData Technologies. Steve is a recognized expert and frequent lecturer on next generation architectures and enterprise security speaking and keynoting at industry and broad market conferences, podcasts, and has authored numerous articles and posts on key technical and strategic topics. He was named one of InfoWorld's Top 25 CTO's, received Executive Mosaic’s Top CTO Executives Award, is a Washington Exec Top Chief Technology Officers to Watch in 2023, was the Vice-Chair of the NSITC/IDESG Security Committee and was a Guest Researcher at NIST’s National Cybersecurity Center of Excellence (NCCoE). He is a fellow at the Center for Advanced Defense Studies and the chair of the INSA Cyber Committee.